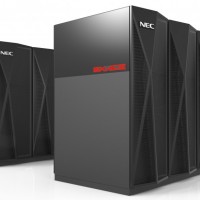
SX-ACE is terminated on Feb 28, 2021.
to 31 March, 2020 | from 1 April, 2020 to 28 February, 2021 | |
---|---|---|
Number of cluster | 3 | 2 |
Number of nodes(total) | 1536 | 1024 |
Number of cores(total) | 6144 | 4096 |
Peak performance(total) | 423TFLOPS | 282TFLOPS |
Vector performance(total) | 393TFLOPS | 262TFLOPS |
Number of memory(total) | 96TB | 64TB |
Storage | 2PB(No change) |
Please keep in mind that SX-ACE service is partly changed as follows after Nov. 30, 2020, due to the replacement work and the contract of SX-ACE system.
- Technical support is NOT available.
- You can NOT "application resource request" and "first application for system use". In case that you use up node-hour of computing resources and storage resources from Dec. 1 to Feb. 28, you cannot add any computing/storage resource. So, we recommend you to add any resource in advance before Nov. 30, 2020.
- You can use shared-use node hour, dedicated-use node, disk(home, ext) which you are currently using, by Feb 28, 2021.
- The additional payment is not necessary for the use of dedicated-use node and disk from Dec.1 to Feb.28.
System overview
The Cybermedia Center has introduced the SX-ACE, which is a “clusterized” vector-typed supercomputer, composed of 3 clusters, each of which is composed of 512 nodes. Each node equips a 4-core multi-core CPU and a 64 GB main memory. These 512 nodes are interconnected on a dedicated and specialized network switch, called the IXS (Internode Crossbar Switch) and forms a cluster. Note that the IXS interconnects 512 nodes with a single lane of a 2-layer fat-tree structure and as a result exhibits 4 GB/s for each direction of input and output between nodes. In the Cybermedia Center, a 2 Peta-byte storage is managed on NEC Scalable Technology File System (ScateFS), a NEC-developed fast distributed and parallel file system, so that it can be accessed from large-scale computing systems including SX-ACE at the Cybermedia Center.
Node performance
Because a node has a multi-core vector-typed processor composed of 4 cores, each of which exhibits a 64 GFlops vector performance, and a 64GB main memory, the vector performance becomes 256 GFlops. On the other hand, the maximum transfer between the CPU and the main memory is 256 GB/s. This fact means that a SX-ACE node achieves a high memory-bandwidth performance of 1 Byte/Flops by taking a higher CPU performance into consideration. Moreover, the SX-ACE is suitable for the purpose of weather/climate and fluid simulation.
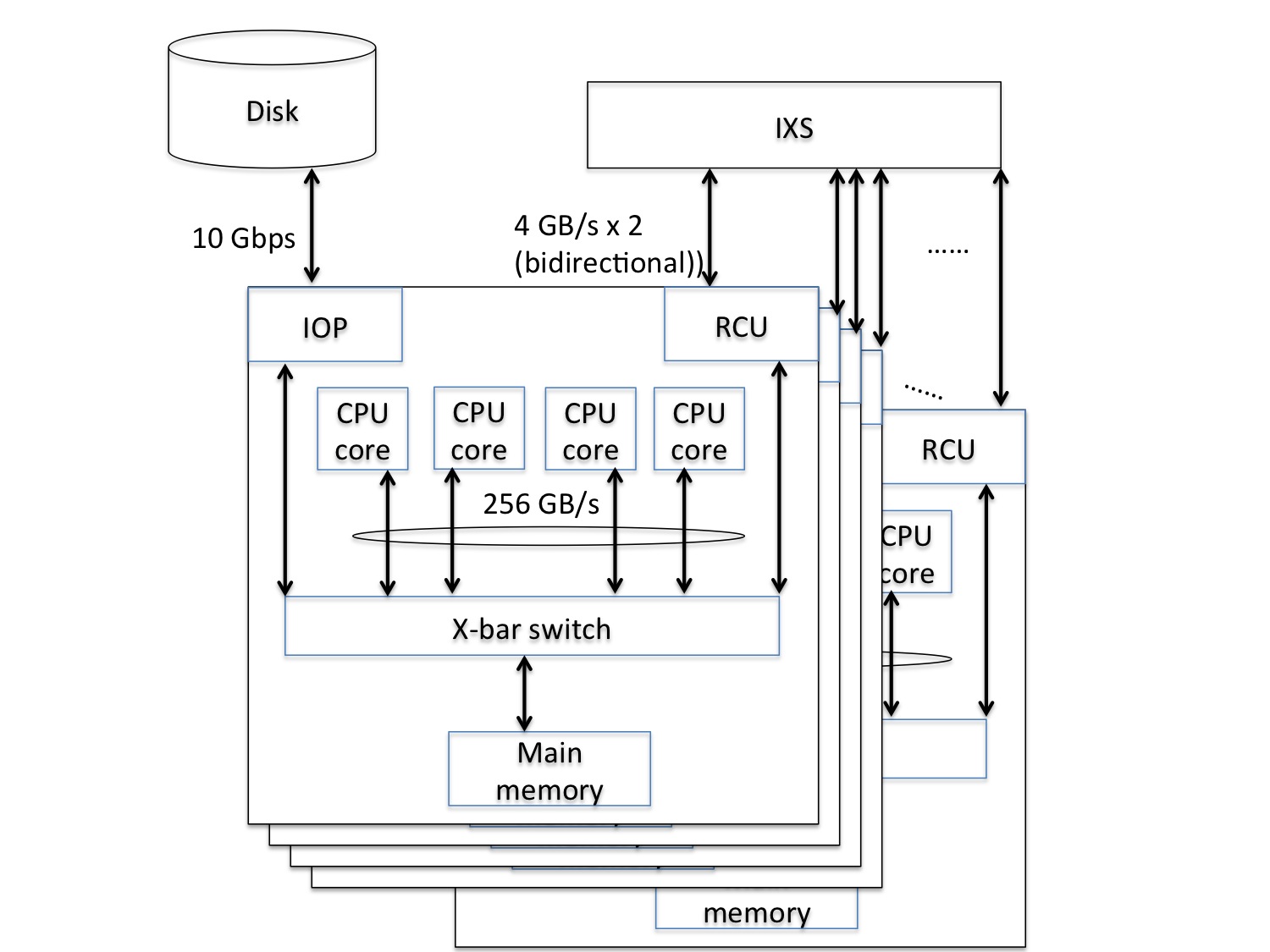
System performance
The Cybermedia Center has introduced the SX-ACE, which is composed of 3 clusters (1536 nodes in total). Therefore, the theoretical peak performance of 1 cluster and of 3 clusters is derived as follows:
SX-ACE
|
|||
Per-node | 1cluster(512node) | Total(3cluster) | |
# of CPU | 1 | 512 | 1536 |
# of core | 4 | 2048 | 6144 |
Performance | 276GFLOPS | 141TFLOPS | 423TFLOPS |
Vector performance | 256GFLOPS | 131TFLOPS | 393TFLOPS |
Main memory | 64GB | 32TB | 96TB |
Storage | 2PB |
Importantly, note that performance is the sum of the vector-typed processor and the scalar processor deployed on SX-ACE. SX-ACE has a 4-core multi-core vector-typed processor and a single scalar processor.
Software
SX-ACE in our center has Super-UX R21.1 installed as the operating system. The Super-UX is based on System V UNIX and provides a high degree of user-experience. Furthermore, this operating system makes full use of the inherent hardware performance of SX-ACE. Because the Super-UX was introduced on SX-8R and SX-9, which the Cybermedia Center had provided as operating system, it is familiar and easy-to-use for experienced users of SX-8R and SX-9.
Also, on the SX-ACE at the CMC, the following software and library, in which performance tuning is applied, are available:
SX-ACE | |
---|---|
Category | Function |
Software for developer | Fortran 95/2003 compiler C/C++ compiler |
MPI library | MPI/SX |
HPF compiler | HPF/SX V2 |
Debugger | dbx/pdbx |
FTRACE | PROGINF/FILEINF FTRACE prof |
Library for numerical calculation | ASL |
Library for statistic calculation | ASLSTAT |
Library for methematic | MathKeisan |
Front-end node | |
---|---|
Category | Function |
Software for developer | Fortran 95/2003 cross-compiler C/C++ cross-compiler Intel Cluster Studio XE |
HPF compiler | HPF/SX V2 cross-compiler |
Debugger | NEC Remote Debugger |
Performance analysis tool | FTRACE NEC Ftrace Viewer |
Visualization software | AVS/ExpressDeveloper |
Software for computational chemistry | Gaussian09 |
An integrated scheduler composed of a JobManipulator and NQSII is used for job management on SX-ACE.
More details about the scheduler are provided here.
Performance tuning on SX-ACE
The SX-ACE is a distributed memory-typed supercomputer interconnecting the small-sized nodes described above, while the vector-typed supercomputers SX-8R and SX-9, provided by the Cybermedia Center, are a shared memory-typed supercomputer where multiple vector-typed CPUs calculate. Under this type of distributed memory-typed supercomputer, computation requires internode communication among nodes with different address spaces. Therefore, for performance tuning on SX-ACE, users are required to have basic knowledge about the internal architecture of nodes, internode structures, and communication characteristics.
Performance tuning techniques on SX-ACE are roughly categorized into two classes of intra-node parallelism (shared-memory parallel processing) and inter-node parallelism (distributed-memory parallel processing). The former parallelism distributes the computational workload to 4 cores on a multi-core vector-typed CPU in SX-ACE, while the latter parallelism distributes the computational workload onto multiple nodes.
Intra-node parallelism (shared-memory parallel processing)
The characteristic of this parallelism is that 4 cores of a multi-core vector-typed CPU shares a 64GB main memory. In general, shared-memory parallel processing is easier in terms of programming and performance tuning than distributed-memory parallel processing. This is true of the SX-ACE so that the intra-node parallelism is easier than the inter-node parallelism.
Representative techniques for Intra-node parallelism on SX-ACE are “Auto parallelism” and “OpenMP”.
This parallelism detects loop structures and instruction sets which the compiler can parallelize. Basically under this parallelism, developers do not need to add and modify their source codes. Developers only need to specify auto-parallelism as a compiler option. The compiler generates an execution module runnable in parallel. In the SX-ACE at the CMC, auto-parallelism is set by feeding a "-P auto" option to the compiler.
If your source code cannot take advantage of auto-parallelism or developers want to turn off the option, they can insert compiler directives to their source code to control auto-parallelism. Detailed information is available here.
OpenMP defines a suite of APIs(Application Programming Interfaces) for shared-memory parallel programming. As the name indicates, OpenMP targets a shared-memory architecture computer. As the following example shows, simply by inserting compiler directives, which are instructions to the compiler, to the source code and then compiling the source code, developers can generate a multi-threaded execution module. Therefore, OpenMP is an easy parallel programming method even the beginner can undertake with ease. The OpenMP can be used in Fortran, C and C++.
ex)1
#pragma omp parallel
{
#pragma omp for
for(i =1; i < 1000; i=i+1) x[i] = y[i] + z[i]; }
ex)2
!$omp parallel
!$omp do
do i=1, 1000
x(i) = y(i) + z(i)
enddo
!$omp enddo
!$omp end parallel
Useful information and TIPS on OpenMP can be available from books and the Internet.
Inter-node parallelism (distributed memory parallel processing)
The characteristic of this parallelism is that it leverages multiple independent memory spaces of distributed nodes, rather than share an identical memory space due to the fact that this parallelism uses multiple nodes. This fact makes this distributed memory parallel processing more difficult than shared-memory parallel processing.
The characteristic of this parallelism is that it leverages multiple independent memory spaces of distributed nodes, rather than share an identical memory space due to the fact that this parallelism uses multiple nodes. This fact makes this distributed memory parallel processing more difficult than shared-memory parallel processing.
A Message Passing Interface provides a set of libraries and APIs for distributed parallel programming based on a message-passing method. Based on considerations of communication patterns that will most likely happen on distributed-memory parallel processing environments, the MPI offers intuitive API sets for peer-to-peer communication and collective communications, in which multiple processes are involved, such as MPI_Bcast and MPI_Reduce. Under MPI parallelism, developers must write a source code by considering data movement and workload distribution among MPI processes. Therefore, MPI is a somewhat more difficult parallelism for beginners. However, developers will learn to write a source code that can run faster than the codes with HPF, once they become intermediate and advanced programmers that can write a source code by considering hardware architecture and other characteristics. Furthermore, MPI has become a de facto standard utilized by many computer simulations and analysis. Moreover, today's computer architecture has become increasingly "Clusterized" and thus, mastering MPI is preferred.
The HPF (High Performance Fortran) is a version of Fortran into which extensions targeting distributed-memory parallel computers is built, which has become an international standard.
Like OpenMP, HPF allows developers to automatically generate an execution module executed by multiple processes simply by inserting compiler directives pertaining to the parallelism of data and processing to source codes. Since the compiler generates instructions related to inter-process communication and synchronization necessary for parallel computation, this parallelism is a relatively easy method for the beginners to realize inter-node parallelism. As the name indicates, HPF is an extension of Fortan and thus, cannot be used in C.
例
!HPF$ PROCESSORS P(4)
!HPF$ DISTRIBUTE (BLOCK) ONTO P :: x,y,z
do i=1, 1000
x(i) = y(i) + z(i)
enddo
SX-9 | SX-ACE | |
---|---|---|
# of CPU(# of core) | 16 CPU | 1 CPU (4core) |
Peak vector performance | 1.6 TFLOPS | 256 TFLOPS (x1/6.4) |
Main memory | 1 TB | 64 GB (x1/16) |